The PyTorch Developer Podcast is a place for the PyTorch dev team to do bite sized (10-20 min) topics about all sorts of internal development topics in PyTorch.
…
continue reading
Scene by Scene is not a traditional movie review podcast. It’s a film discussion podcast with two independent filmmakers fascinated by the filmmaking process. Every other week, Joe and Justin break down the elements of one movie to better understand how films are made, what makes a “good” one, and what makes a “bad” one. After examining the film's story and themes, visual technique, and historical, cultural, or personal significance, they discuss what they've learned.
…
continue reading
In this podcast, we will focus on making your life better, a plus-up, by achieving success in the three core components of life: Personal, Finance, and Career. For over 15 years, I have been in many roles, such as financial executive, board member of many non-profits, career mentor, education curriculum director, and online columnist. Regardless of what title I hold, I will always view myself as a life coach who dedicated to helping others with personal and professional skills. IG: @lifeplus ...
…
continue reading
Jesse Felder worked for the largest firm on Wall Street, co-founded a multi-billion-dollar hedge fund firm and has been active in the markets for over 20 years. This podcast is his journey to talk with a number of superinvestors who have been an inspiration to him in an effort to understand what makes them so successful in the financial markets and in life.
…
continue reading
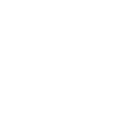
1
That Day, on the Beach (1983) | Dir. Edward Yang
2:49:03
2:49:03
Play later
Play later
Lists
Like
Liked
2:49:03
In this episode, we discuss Edward Yang’s That Day, on the Beach, including the theme of tradition vs modernity, the film’s structure, and the techniques used to transition into flashbacks. Supplemental Material: Nova Media's That Day, on the Beach Blu-ray That Day, on the Beach (Internet Archive) That Day, on the Beach (rarefilmm) Edward Yang (Con…
…
continue reading
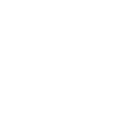
1
Shadows (1959) | Dir. John Cassavetes
2:24:25
2:24:25
Play later
Play later
Lists
Like
Liked
2:24:25
In this episode, we discuss John Cassavetes' Shadows including how the film grew from Cassavetes’ personal experiences, Cassavetes’ use of improvisation, and techniques used to communicate information about characters and their relationship with one another. See where Shadows is available to watch. Supplemental Material: The Criterion Collection's …
…
continue reading
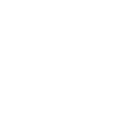
1
Killer Klowns from Outer Space (1988) | Dir. Stephen Chiodo
2:53:17
2:53:17
Play later
Play later
Lists
Like
Liked
2:53:17
Trash Cinema Month is Back! In this episode, we discuss Stephen Chiodo's Killer Klowns from Outer Space, including the killing of dogs in movies, how it compares to 1950s creature features, and the lack of understandable klown motivation. See where Killer Klowns from Outer Space is available to watch. Supplemental Material: Killer Klowns from Outer…
…
continue reading
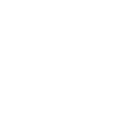
1
Nightmare Sisters (1988) | Dir. David DeCoteau
2:12:50
2:12:50
Play later
Play later
Lists
Like
Liked
2:12:50
Trash Cinema Month is Back! In this episode, we discuss David DeCoteau's Nightmare Sisters, including the lack of horror elements, how the film’s short schedule affected the film grammar, and how we found inspiration through the film. See where Nightmare Sisters is available to watch. Supplemental Material: Vinegar Syndrome's Nightmare Sisters Blu-…
…
continue reading
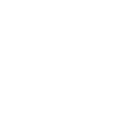
1
Cléo from 5 to 7 (1962) | Dir. Agnès Varda
1:44:03
1:44:03
Play later
Play later
Lists
Like
Liked
1:44:03
In this episode, we discuss Agnès Varda's Cléo from 5 to 7, including how Cléo goes from viewed to viewer, the techniques used to communicate Cléo's change, and how Varda balances fantasy and reality. See where Cléo from 5 to 7 is available to watch. Supplemental Material: The Criterion Collection's The Complete Films of Agnès Varda Blu-ray Cléo fr…
…
continue reading
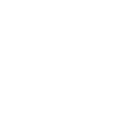
1
Claire's Knee (1970) | Dir. Eric Rohmer
2:04:53
2:04:53
Play later
Play later
Lists
Like
Liked
2:04:53
In this episode, we discuss Eric Rohmer's Claire's Knee, including how Jerôme’s actions contradict his words, the characters’ lack of maturity, and how the blocking communicates character relationships. See where Claire's Knee is available to watch. Supplemental Material: The Criterion Collection's Six Moral Tales Blu-ray Video Essay: How the Frenc…
…
continue reading
TORCH_TRACE and tlparse are a structured log and log parser for PyTorch 2. It gives useful information about what code was compiled and what the intermediate build products look like.By PyTorch
…
continue reading
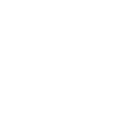
1
American Movie (1999) | Dir. Chris Smith
2:24:07
2:24:07
Play later
Play later
Lists
Like
Liked
2:24:07
In this episode, we discuss Chris Smith's American Movie, including Mark’s performative side, Mark and Mike’s friendship, and the ways we see ourselves in Mark. See where American Movie is available to watch. Supplemental Material: American Movie Blu-ray Coven (Dir. Mark Borchardt) I Blow Up (Dir. Mark Borchardt) Mike Schank's Music Filmmaker Mark …
…
continue reading
Higher order operators are a special form of operators in torch.ops which have relaxed input argument requirements: in particular, they can accept any form of argument, including Python callables. Their name is based off of their most common use case, which is to represent higher order functions like control flow operators. However, they are also u…
…
continue reading
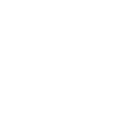
1
Giuseppe Makes a Movie (2014) | Dir. Adam Rifkin
2:05:57
2:05:57
Play later
Play later
Lists
Like
Liked
2:05:57
In this episode, we discuss Adam Rifkin's Giuseppe Makes a Movie, including Giuseppe’s filmmaking process, how it inspires us, and the importance of documenting a community that no longer exists. See where Giuseppe Makes a Movie is available to watch. Supplemental Material: Giuseppe Makes a Movie Official Website Cinelicious Pics' Giuseppe Makes a …
…
continue reading
The post-grad FX passes in Inductor run after AOTAutograd has functionalized and normalized the input program into separate forward/backward graphs. As such, they generally can assume that the graph in question is functionalized, except for some mutations to inputs at the end of the graph. At the end of post-grad passes, there are special passes th…
…
continue reading
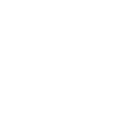
1
Three Colors: Red (1994) | Dir. Krzysztof Kieślowski
2:07:17
2:07:17
Play later
Play later
Lists
Like
Liked
2:07:17
In this episode, we discuss Krzysztof Kieślowski's Three Colors: Red, including its connections to Blue and White, the film's telephone motif, and the way Kieślowski’s camera conveys connection and disconnection. See where Three Colors: Red is available to watch. Supplemental Material: The Criterion Collection's Three Colors Blu-ray Curzon's Three …
…
continue reading
CUDA graph trees are the internal implementation of CUDA graphs used in PT2 when you say mode="reduce-overhead". Their primary innovation is that they allow the reuse of memory across multiple CUDA graphs, as long as they form a tree structure of potential paths you can go down with the CUDA graph. This greatly reduced the memory usage of CUDA grap…
…
continue reading
The min-cut partitioner makes decisions about what to save for backwards when splitting the forward and backwards graph from the joint graph traced by AOTAutograd. Crucially, it doesn't actually do a "split"; instead, it is deciding how much of the joint graph should be used for backwards. I also talk about the backward retracing problem.…
…
continue reading
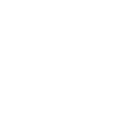
1
Three Colors: White (1994) | Dir. Krzysztof Kieślowski
1:21:16
1:21:16
Play later
Play later
Lists
Like
Liked
1:21:16
In this episode, we discuss Krzysztof Kieślowski's Three Colors: White, including the strong opening sequence, its connections to Three Colors: Blue, and whether the film effectively handles the theme of equality. See where Three Colors: White is available to watch. Supplemental Material: The Criterion Collection's Three Colors Blu-ray Curzon's Thr…
…
continue reading
AOTInductor is a feature in PyTorch that lets you export an inference model into a self-contained dynamic library, which can subsequently be loaded and used to run optimized inference. It is aimed primarily at CUDA and CPU inference applications, for situations when your model export once to be exported once while your runtime may still get continu…
…
continue reading
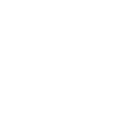
1
Three Colors: Blue (1993) | Dir. Krzysztof Kieślowski
1:58:07
1:58:07
Play later
Play later
Lists
Like
Liked
1:58:07
In this episode, we discuss Krzysztof Kieślowski's Three Colors: Blue, including possible interpretations of the film’s abstract moments, the depiction of loss and grief, and Juliette Binoche's internal performance. See where Three Colors: Blue is available to watch. Supplemental Material: The Criterion Collection's Three Colors Blu-ray Curzon's Th…
…
continue reading
Tensor subclasses allow you to add extend PyTorch with new types of tensors without having to write any C++. They have been used to implement DTensor, FP8, Nested Jagged Tensor and Complex Tensor. Recent work by Brian Hirsh means that we can compile tensor subclasses in PT2, eliminating their overhead. The basic mechanism by which this compilation …
…
continue reading
Compiled autograd is an extension to PT2 that permits compiling the entirety of a backward() call in PyTorch. This allows us to fuse accumulate grad nodes as well as trace through arbitrarily complicated Python backward hooks. Compiled autograd is an important part of our plans for compiled DDP/FSDP as well as for whole-graph compilation.…
…
continue reading
We discuss some extension points for customizing PT2 behavior across Dynamo, AOTAutograd and Inductor.By PyTorch
…
continue reading
Define-by-run IR is how Inductor defines the internal compute of a pointwise/reduction operation. It is characterized by a function that calls a number of functions in the 'ops' namespace, where these ops can be overridden by different handlers depending on what kind of semantic analysis you need to do. The ops Inductor supports include regular ari…
…
continue reading
Traditionally, unsigned integer support in PyTorch was not great; we only support uint8. Recently, we added support for uint16, uint32 and uint64. Bare bones functionality works, but I'm entreating the community to help us build out the rest. In particular, for most operations, we plan to use PT2 to build anything else. But if you have an eager ker…
…
continue reading
Inductor IR is an intermediate representation that lives between ATen FX graphs and the final Triton code generated by Inductor. It was designed to faithfully represent PyTorch semantics and accordingly models views, mutation and striding. When you write a lowering from ATen operators to Inductor IR, you get a TensorBox for each Tensor argument whi…
…
continue reading
I talk about VariableTracker in Dynamo. VariableTracker is Dynamo's representation of the Python. I talk about some recent changes, namely eager guards and mutable VT. I also tell you how to find the functionality you care about in VariableTracker (https://docs.google.com/document/d/1XDPNK3iNNShg07jRXDOrMk2V_i66u1hEbPltcsxE-3E/edit#heading=h.i6v7gq…
…
continue reading
We’re sorry for the delay on this episode, but we hope you enjoy the looser discussion. We’ll be back on March 1st, discussing Krzysztof Kieślowski’s Three Colors: Blue. In this episode, we discuss our favorite films featured on the podcast. We also discuss the best and worst films we watched in 2023, lessons learned, and our 2024 film resolutions.…
…
continue reading
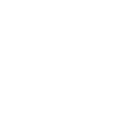
1
Christmas, Again (2014) | Dir. Charles Poekel
1:46:59
1:46:59
Play later
Play later
Lists
Like
Liked
1:46:59
In this episode, we discuss Charles Poekel's Christmas, Again, including Poekel's extensive research, Kentucker Audley's naturalistic performance, and the film's lack of traditional exposition. See where Christmas, Again is available to watch. Supplemental Material: Factory 25's Christmas, Again Blu-ray Christmas, Again Kickstarter Christmas, Again…
…
continue reading
This episode features discussion of suicide. The 988 Lifeline provides 24/7, free and confidential support for people in distress, prevention and crisis resources for you or your loved ones, and best practices for professionals in the United States. https://988lifeline.org/ In this episode, we discuss Edson Oda's Nine Days, including multiple inter…
…
continue reading
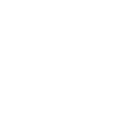
1
After Life (1998) | Dir. Hirokazu Kore-eda
1:23:12
1:23:12
Play later
Play later
Lists
Like
Liked
1:23:12
In this episode, we discuss Hirokazu Kore-eda's After Life, including the film's documentary aesthetic, how Kore-eda communicates information visually, and the way exposition is made engaging. See where After Life is available to watch. Supplemental Material: The Criterion Collection's After Life Blu-ray BFI's After Life Blu-ray After Life: In Memo…
…
continue reading
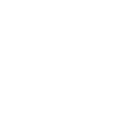
1
The Descent (2005) | Dir. Neil Marshall
1:56:12
1:56:12
Play later
Play later
Lists
Like
Liked
1:56:12
In this episode, we discuss Neil Marshall's The Descent, including the film’s lighting, the mistakes made in the first act, and whether the creatures help or harm the film. See where The Descent is available to watch. Supplemental Material: The Descent Blu-ray Additional Audio Sources: The Descent Trailer "The Descent: Beneath the Scenes" on The De…
…
continue reading
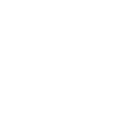
1
Prince of Darkness (1987) | Dir. John Carpenter
1:52:56
1:52:56
Play later
Play later
Lists
Like
Liked
1:52:56
In this episode, we discuss John Carpenter's Prince of Darkness, including the screenplay's missteps, the effective use of insects, and Carpenter's visual techniques. See where Prince of Darkness is available to watch. Supplemental Material: Scream Factory's Prince of Darkness Blu-ray John Carpenter on Managing to "Survive" Hollywood (Variety Inter…
…
continue reading
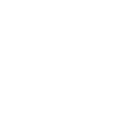
1
It Comes at Night (2017) | Dir. Trey Edward Shults
1:36:26
1:36:26
Play later
Play later
Lists
Like
Liked
1:36:26
In this episode, we discuss Trey Edward Shults' It Comes at Night, including the film's marketing, our interpretations of "it", and the film's use of traditional horror conventions and iconography. See where It Comes at Night is available to watch. Supplemental Material: It Comes at Night Blu-ray Anatomy of a Scene: Trey Edward Shults Narrates a Sc…
…
continue reading
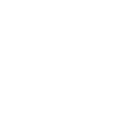
1
Possession (1981) | Dir. Andrzej Żuławski
1:41:37
1:41:37
Play later
Play later
Lists
Like
Liked
1:41:37
In this episode, we discuss Andrzej Żuławski’s Possession, including our interpretations of the film's creature and doppelgängers, the expressive actor blocking, and the abrasive (yet successful) performances. See where Possession is available to watch. Supplemental Material: Mondo Vision's Possession Blu-ray Additional Audio Sources: Possession Of…
…
continue reading
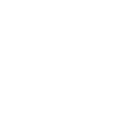
1
Synecdoche, New York (2008) | Dir. Charlie Kaufman
1:57:21
1:57:21
Play later
Play later
Lists
Like
Liked
1:57:21
In this episode, we discuss Charlie Kaufman’s Synecdoche, New York, including the lack of subtlety, the execution of numerous themes and ideas, and whether the film encapsulates the human experience. See where Synecdoche, New York is available to watch. Supplemental Material: Synecdoche, New York Blu-ray Charlie Kaufman | BAFTA Screenwriters’ Lectu…
…
continue reading
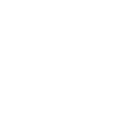
1
Aguirre, the Wrath of God (1972) | Dir. Werner Herzog
1:58:25
1:58:25
Play later
Play later
Lists
Like
Liked
1:58:25
In this episode, we discuss Werner Herzog's Aguirre, the Wrath of God, including Herzog's process, the unconventional storytelling, and the masterful approach to perspective. See where Aguirre, the Wrath of God is available to watch. Supplemental Material: Shout! Factory's Aguirre, the Wrath of God Blu-ray Werner Herzog's MasterClass Werner Herzog:…
…
continue reading
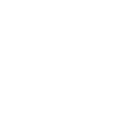
1
No Bears (2022) | Dir. Jafar Panahi
1:40:46
1:40:46
Play later
Play later
Lists
Like
Liked
1:40:46
In this episode, we discuss Jafar Panahi's No Bears, including the self-reflective themes, Panahi's focus on women in Iranian society, and how Panahi captures the documentary aesthetic. See where No Bears is available to watch. Supplemental Material: Jafar Panahi's Miami Film Festival Precious Gem Award Acceptance Speech Ramin Bahrani on No Bears J…
…
continue reading
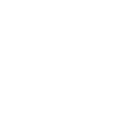
1
Bless Their Little Hearts (1984) | Dir. Billy Woodberry
1:46:06
1:46:06
Play later
Play later
Lists
Like
Liked
1:46:06
In this episode, we discuss Billy Woodberry's Bless Their Little Hearts, including the film's expertly crafted opening, the theme of masculinity, and the film's place within the L.A. Rebellion film movement. See where Bless Their Little Hearts is available to watch. Supplemental Material: Milestone Films' Bless Their Little Hearts DVD UCLA Library …
…
continue reading
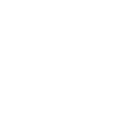
1
Miami Connection (1987) | Dir. Richard Park & Y.K. Kim
1:48:36
1:48:36
Play later
Play later
Lists
Like
Liked
1:48:36
In this episode, we discuss Richard Park & Y.K. Kim's Miami Connection, including Kim’s aspirations for the film, the unsuccessful execution of fight scenes, and why every character is an orphan. See where Miami Connection is available to watch. Supplemental Material: Vinegar Syndrome's Miami Connection 4K Ultra HD + Blu-ray Vice Outsider | TaeKwon…
…
continue reading
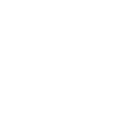
1
#57: Asif Suria On The Virtues Of Event-Driven Investing
1:07:56
1:07:56
Play later
Play later
Lists
Like
Liked
1:07:56
This episode is long overdue. I've been following the work of Asif Suria for over a decade now and it has proven to be one the best sources of investment ideas I have come across. Asif tracks and writes about everything from predictive insider trading patterns to "ubercannibals," or companies that consistently use excess free cash flow to buyback s…
…
continue reading
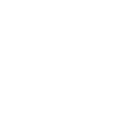
1
Hard Ticket to Hawaii (1987) | Dir. Andy Sidaris
2:07:17
2:07:17
Play later
Play later
Lists
Like
Liked
2:07:17
In this episode, we discuss Andy Sidaris' Hard Ticket to Hawaii, including the nonsensical narrative and character decisions, the lazy action filmmaking, and Sidaris’ filmmaker trademarks. See where Hard Ticket to Hawaii is available to watch. Supplemental Material: Andy Sidaris Official Website Mill Creek's Hard Ticket to Hawaii Blu-ray Naked Trut…
…
continue reading
In this episode, we introduce our June film series, Trash Cinema Month, including the definition of “Trash Cinema”, what makes a “Trash Film”, and the value of watching and discussing “Trash Cinema”. Join us for our first Trash Cinema Month selection, Hard Ticket to Hawaii. Supplemental Material: Trash Cinema: The Lure of the Low (Shortcuts Series)…
…
continue reading
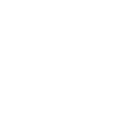
1
#56: Joseph Wang On How The Fed Forever Altered The Investing Landscape
1:03:03
1:03:03
Play later
Play later
Lists
Like
Liked
1:03:03
My guest for this episode is Joseph Wang. Joseph is one of the most knowledgeable people I’ve come across when it comes to the world of central banking and how it intersects with markets. His experience on the open markets desk at the New York Fed gives him unique insight into the inner workings of the financial system and how it has evolved in rec…
…
continue reading
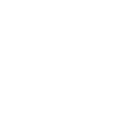
1
Aftersun (2022) | Dir. Charlotte Wells
1:58:50
1:58:50
Play later
Play later
Lists
Like
Liked
1:58:50
In this episode, we discuss Charlotte Wells' Aftersun, including the numerous ideas and themes presented, the brave filmmaking choices, and our differing interpretations of the film's final moments. See where Aftersun is available to watch. Supplemental Material: Charlotte Wells' Short Films Aftersun Screenplay Charlotte Wells Interview with Film a…
…
continue reading
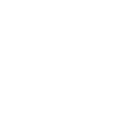
1
#55: Ben Hunt On The Big 'Bust Out'
1:06:18
1:06:18
Play later
Play later
Lists
Like
Liked
1:06:18
My guest for this episode is Ben Hunt. This is Ben’s second appearance on the podcast. I had the pleasure of first interviewing him a few years ago and if you are not familiar with his work, I encourage you to go listen to that episode in which he discusses his background and the key concepts he utilizes in analyzing markets. It’s not at all necess…
…
continue reading
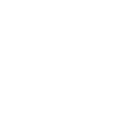
1
Shiva Baby (2020) | Dir. Emma Seligman
1:24:03
1:24:03
Play later
Play later
Lists
Like
Liked
1:24:03
In this episode, we discuss Emma Seligman's Shiva Baby, including how the feature compares to the short, the tightrope walk between comedy and anxiety, and how the film grammar places the audience into Danielle’s perspective. See where Shiva Baby is available to watch. Supplemental Material: Utopia's Shiva Baby Blu-ray Making It: Women in Film | Ex…
…
continue reading
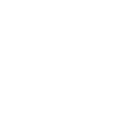
1
The Handmaiden (2016) | Dir. Park Chan-wook
1:44:07
1:44:07
Play later
Play later
Lists
Like
Liked
1:44:07
In this episode, we discuss Park Chan-wook’s The Handmaiden, including the screenplay's masterful use of setup and payoff, the film's relationship to the rest of Park's filmography, and Park's filmmaking process. See where The Handmaiden is available to watch. Supplemental Material: Curzon's The Handmaiden Blu-ray The Handmaiden: Why Costume Design…
…
continue reading
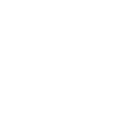
1
Rebels of the Neon God (1992) | Dir. Tsai Ming-liang
1:35:11
1:35:11
Play later
Play later
Lists
Like
Liked
1:35:11
In this episode, we discuss Tsai Ming-liang’s Rebels of the Neon God, including its similarities to Edward Yang's The Terrorizers, Hsiao-Kang's multi-layered motivations, and Tsai's skill at introducing/establishing characters. See where Rebels of the Neon God is available to watch. Supplemental Material: Big World Pictures' Rebels of the Neon God …
…
continue reading
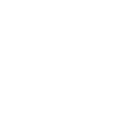
1
The Terrorizers (1986) | Dir. Edward Yang
1:41:04
1:41:04
Play later
Play later
Lists
Like
Liked
1:41:04
After debate over the title, we discuss Edward Yang’s The Terrorizers, including the unconventional filmmaking, the possible interpretations of the ending, and who is/are the terrorizer/s. See where The Terrorizers is available to watch. Supplemental Material: Edward Yang (Contemporary Film Directors) (Book) It's All Over: Edward Yang’s The Terrori…
…
continue reading
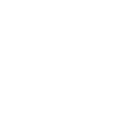
1
Mulholland Drive (2001) | Dir. David Lynch
1:44:52
1:44:52
Play later
Play later
Lists
Like
Liked
1:44:52
In this episode, we discuss David Lynch’s Mulholland Drive, including the film's journey from television pilot to the silver screen, the many possible interpretations, and what it means to be Lynchian. See where Mulholland Drive is available to watch in the US | UK. Supplemental Material: The Criterion Collection's Mulholland Dr. (Blu-ray) Lynch on…
…
continue reading
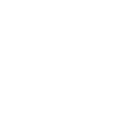
1
Metropolis (1927) | Dir. Fritz Lang
1:38:21
1:38:21
Play later
Play later
Lists
Like
Liked
1:38:21
In our debut episode, we discuss Fritz Lang’s Metropolis, including the restoration that produced this most complete version to date, the culture that Metropolis influenced, and Metropolis’ status as a masterpiece. See where Metropolis is available to watch in the US | UK. Supplemental Material: Kino Lorber's The Complete Metropolis (Blu-ray) Eurek…
…
continue reading
In this episode, we introduce ourselves, explain the show’s format, and discuss what we hope to accomplish with Scene by Scene. If you'd like to support the show, subscribe to the podcast and rate/review on Apple Podcasts, Spotify, or wherever you listen to your podcasts. If you have any thoughts, comments, or questions about the show, you can emai…
…
continue reading