Join Sophia V. Prater on a dive into the weeds on UX systems, information architecture, human psychology, and simplifying the complex. Support this podcast: https://podcasters.spotify.com/pod/show/ooux/support
…
continue reading
Real life code talk between two working developers.
…
continue reading
The world of computer programming is vast in scope. There are literally thousands of topics to cover and no one person could ever reach them all. One of the goals of the Coding Blocks podcast is to introduce a number of these topics to the audience so they can learn during their commute or while cutting the grass. We will cover topics such as best programming practices, design patterns, coding for performance, object oriented coding, database design and implementation, tips, tricks and a who ...
…
continue reading
Welcome to Control Intelligence, a ControlDesign.com podcast that goes deep inside the automation and technology that machine builders, system integrators and end users rely on to keep production humming efficiently
…
continue reading
The Smalltalk programming language is not only the first pure object oriented language, but has been the birthplace for many of today's best practices in software development including agile methods, design patterns, unit testing, refactoring and incremental development. In the Smalltalk Reflections podcast, David Buck and Craig Latta guide you through the world of Smalltalk covering topics from basic object oriented principles to software testing, version control and deployment techniques. ...
…
continue reading
This is a feed of pages for iversen1450149
…
continue reading
A podcast hosted by Kathryn Hodge and Robyn Silber that aims to introduce others to coding, demystify core programming concepts, provide tips for success, and start more conversations around important topics in technology.
…
continue reading
The moths of programming are ideas in the software engineering industry that have become pervasive due to virality rather than reality.
…
continue reading
Not your average fitspo – talking all things fitness, self growth, and business with an authentic yet authoritative approach.
…
continue reading
As it concerns the racial history of our country, are the objects in the mirror closer than they appear or not? Objects In The Mirror podcast asks this question as listeners hear firsthand accounts of those who lived during the segregation and early desegregation eras.
…
continue reading
24 Minutes of UX is a podcast of the people, by the people, for the people. The format is a combination of an after-work discussion, a 1-on-1 chat, a global community platform, and a coaching session. Episodes typically feature a Seeker and a Giver. The Seeker is a person seeking advice on a specific topic within the domain of UX. The Giver is here for sharing experiences about “what I wish I had known when I started with this topic”. Both the Seeker and the Giver can be senior or junior - w ...
…
continue reading
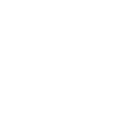
1
Object-oriented programming or ladder logic?
3:47
3:47
Play later
Play later
Lists
Like
Liked
3:47
In this episode of Control Intelligence, written by contributing editor Tobey Strauch, editor in chief Mike Bacidore discusses object-oriented programming in PLCs.By controldesign
…
continue reading
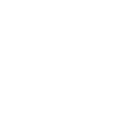
1
5 use cases for the uninterruptible power supply
7:08
7:08
Play later
Play later
Lists
Like
Liked
7:08
In this episode of Control Intelligence, written by contributing editor Joey Stubbs, editor in chief Mike Bacidore shares what uninterruptible power supplies are.By controldesign
…
continue reading
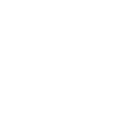
1
Wireless HMI enables endless control and monitoring
6:41
6:41
Play later
Play later
Lists
Like
Liked
6:41
In this episode of Control Intelligence, written by contributing editor Jeremy Pollard, editor in chief Mike Bacidore discusses the uses of wireless HMI.By controldesign
…
continue reading
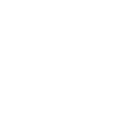
1
October 2024 industry news: acquisitions, new facilities and AI
14:39
14:39
Play later
Play later
Lists
Like
Liked
14:39
In this episode of Control Intelligence, managing editor Anna Townshend shares machine builder and system integrator news highlights from the month of October.By controldesign
…
continue reading
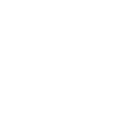
1
Fiberglass, ABS or recycled plastic materials create unique enclosure troubleshooting scenarios
6:39
6:39
Play later
Play later
Lists
Like
Liked
6:39
In this episode of Control Intelligence, written by contributing editor Joey Stubbs, editor in chief Mike Bacidore shares how to troubleshoot electrical noise in composite enclosures.By controldesign
…
continue reading
https://venmo.com/bewellAVLBy Daniel Coulbourne & Caleb Porzio
…
continue reading
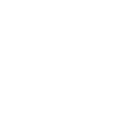
1
The evolution of the programmable automation controller
6:36
6:36
Play later
Play later
Lists
Like
Liked
6:36
In this episode of Control Intelligence, written by contributing editor Jeremy Pollard, editor in chief Mike Bacidore differentiates between programmable automation controllers and programmable logic controllers.By controldesign
…
continue reading
Well, this is awkward. Coding Blocks is signing out for now, in this episode we’ll talk about what’s happening and why. We have had an amazing run, far better than we ever expected. Also, Joe recommends 50 games, Allen goes for the gold, and Outlaw is totally normal. (And we’re not crying you’re crying!) Thank you for the support over the last 11 (…
…
continue reading
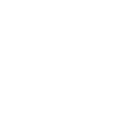
1
September 2024 industry news: reports, associations and new leaders
17:33
17:33
Play later
Play later
Lists
Like
Liked
17:33
In this episode of Control Intelligence, managing editor Anna Townshend shares machine builder and system integrator news highlights from the month of September.By controldesign
…
continue reading
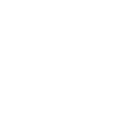
1
065 - Why I'm Closing the Doors on the Self-Paced OOUX Masterclass
25:59
25:59
Play later
Play later
Lists
Like
Liked
25:59
The Self-Paced OOUX Masterclass is coming off the shelf at midnight on Friday, September 27, 2024. In this solo episode of the podcast, Sophia talks about why she's taking the course off the market, when it will be back, and why you should get this "life-changing," "mind blowing," "career-saving" course today before it's gone, plus two big lessons …
…
continue reading
In this episode of Control Intelligence, written by contributing editor Rick Rice, editor in chief Mike Bacidore discusses how to protect HMIs in hazardous environments.By controldesign
…
continue reading
Darkmode VSOPBy Daniel Coulbourne & Caleb Porzio
…
continue reading
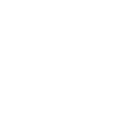
1
How RFID can be used in real-time inventory management, seamless asset tracking, quality control and streamlined production processes
7:37
7:37
Play later
Play later
Lists
Like
Liked
7:37
In this episode of Control Intelligence, written by contributing editor Joey Stubbs, editor in chief Mike Bacidore shares four use cases of radio-frequency identification.By controldesign
…
continue reading
In this episode of Control Intelligence, written by contributing editor Jeremy Pollard, editor in chief Mike Bacidore discusses how industrial networks differ from commercial networks.By controldesign
…
continue reading
Kids have it the worst, dude.By Daniel Coulbourne & Caleb Porzio
…
continue reading
In 2025, AVG Automation, parent company of EZAutomation, a midwestern U.S. manufacturer and factory-direct purveyor of industrial automation systems and components, will celebrate 50 years since its founding in 1975. The automation technology landscape has changed dramatically since that time, but many of the underlying industry needs remain unchan…
…
continue reading
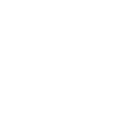
1
Things to Know when Considering Multi-Tenant or Multi-Threaded Applications
1:58:45
1:58:45
Play later
Play later
Lists
Like
Liked
1:58:45
For the full show notes head over to: https://www.codingblocks.net/episode241By Michael Outlaw, Allen Underwood, Joe Zack
…
continue reading
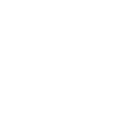
1
August 2024 industry news: events, standards and executive appointments
17:17
17:17
Play later
Play later
Lists
Like
Liked
17:17
In this episode of Control Intelligence, managing editor Anna Townshend shares machine builder and system integrator news highlights from the month of August.By controldesign
…
continue reading
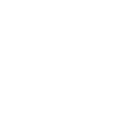
1
Embracing cybersecurity with Nozomi Networks
5:03
5:03
Play later
Play later
Lists
Like
Liked
5:03
In this episode of Control Intelligence, written by contributing editor Tobey Strauch, editor-in-chief Mike Bacidore discusses cybersecurity-enabled controllers.By controldesign
…
continue reading
Grab your headphones because it's water cooler time! In this episode we're catching up on feedback, putting our skills to the test, and wondering what we're missing. Plus, Allen's telling it how it is, Outlaw is putting it all together and Joe is minding the gaps! View the full show notes here: https://www.codingblocks.net/episode240 Reviews Thank …
…
continue reading
Things are in full swing. There are many things we cannot say. We are both slammed trying to get everything done for Laracon. Which led to this not being put out very quickly.By Daniel Coulbourne & Caleb Porzio
…
continue reading
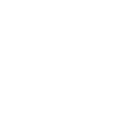
1
Protecting and serving data with sensing devices
5:44
5:44
Play later
Play later
Lists
Like
Liked
5:44
In this Control Intelligence podcast, written by contributing editor Jeremy Pollard, editor-in-chief Mike Bacidore explores networking capabilities with sensing devices.By controldesign
…
continue reading
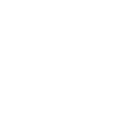
1
064 - The Three Transformations Most Designers Crave
34:59
34:59
Play later
Play later
Lists
Like
Liked
34:59
In this solo episode of the podcast, Sophia asks, what is the point of OOUX? What are the things people get out of OOUX? Sophia outlines the three glorious transformations that OOUX provides to the weary UX professional. This podcast is for people who are wondering if they should dive deeper into the world of Object-Oriented UX. LINKS: Get started …
…
continue reading
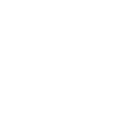
1
How RFID continues to evolve in industrial applications
18:41
18:41
Play later
Play later
Lists
Like
Liked
18:41
Pepperl+Fuchs’ Michael McCabe explains standardized tags, data transfer rates, capabilities, security and integrationBy controldesign
…
continue reading
For the full show notes please visit: https://www.codingblocks.net/episode239By Michael Outlaw, Allen Underwood, Joe Zack
…
continue reading
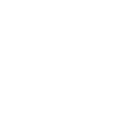
1
July 2024 industry news: certifications, leaders and obituaries
20:47
20:47
Play later
Play later
Lists
Like
Liked
20:47
In this episode of Control Intelligence, Anna Townshend shares machine builder and system integrator news highlights from July 2024.By controldesign
…
continue reading
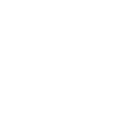
1
When to consider Arduino, Raspberry Pi and other microcontrollers
6:31
6:31
Play later
Play later
Lists
Like
Liked
6:31
In this episode of Control Intelligence, written by contributing editor Jeremy Pollard, editor-in-chief Mike Bacidore explains how new frontiers in machine control have their upsides and their limitations.By controldesign
…
continue reading
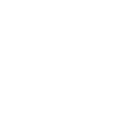
1
063 - Upgrading OOUX and ORCA and Why the Masterclass is going on Pause
37:17
37:17
Play later
Play later
Lists
Like
Liked
37:17
There are big changes coming to the world of OOUX. In this episode of the podcast, Sophia talks about bumping the ORCA process up from 15 to 16 steps. She also discusses the upcoming Cohort 10, which will mark the tenth anniversary of OOUX! And to celebrate that milestone she has devised four new bonuses for this cohort. Plus, why we will be puttin…
…
continue reading
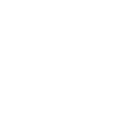
1
Advances in stepper motors to provide precise motion control
7:11
7:11
Play later
Play later
Lists
Like
Liked
7:11
In this episode of Control Intelligence, written by contributing editor Joey Stubbs, editor-in-chief Mike Bacidore discusses what makes stepper motors more energy efficient and reliable.By controldesign
…
continue reading
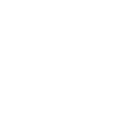
1
How system integrators are using digital tools
6:35
6:35
Play later
Play later
Lists
Like
Liked
6:35
In this episode of Control Intelligence, editor-in-chief Mike Bacidore shares highlights from a panel discussion that took place at the CSIA Exchange.By controldesign
…
continue reading
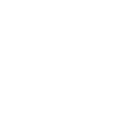
1
AI, Blank Pages, and Client Libraries...oh my!
1:47:21
1:47:21
Play later
Play later
Lists
Like
Liked
1:47:21
It's Water Cooler Time! We've got a variety of topics today, and also Outlaw's lawyering up, Allen can read QR codes now, and Joe is looking at second careers. View the full show notes here: https://www.codingblocks.net/episode238 News As always, thank you for leaving us a review – we really appreciate them! Almazkun, vassilbakalov, DzikijSver Atla…
…
continue reading
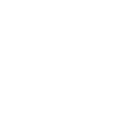
1
What are your sensing options in industrial manufacturing?
7:48
7:48
Play later
Play later
Lists
Like
Liked
7:48
In this episode of Control Intelligence, written by contributing editor Charles Palmer, editor-in-chief Mike Bacidore discusses lasers and other position sensing.By controldesign
…
continue reading
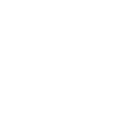
1
062 - Proof that OOUX Works with Kate Redfern and Philippe Fara
1:14:19
1:14:19
Play later
Play later
Lists
Like
Liked
1:14:19
Kate Redfern is a Senior UX Consultant at Manifesto, a digital experience agency that works with clients in the non-profit and government space. Philippe Fara is the Digital Experience Manager for Renfrewshire Council. In this episode of the podcast, Sophia, Kate, and Philippe discuss using the ORCA process to raise user competency scores for the R…
…
continue reading
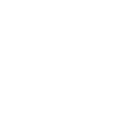
1
Human-Centered Agile with Daniel Boos, Joe Montalbano and Brad Lehman
29:38
29:38
Play later
Play later
Lists
Like
Liked
29:38
The book Human-Centered Agile by Joe Montalbano and Brad Lehman offers strategies to combine Agile and UX. Daniel Boos, co-lead of UX practices and Swiss Railways read the book, but still has questions, for example about organizations where agile may not fit, shared services teams, scaling agile, or the plethora of Ops that are emerging. Luckily Jo…
…
continue reading
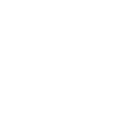
1
June 2024 industry news: new machines, anniversaries and name changes
12:11
12:11
Play later
Play later
Lists
Like
Liked
12:11
In this episode of Control Intelligence, managing editor Anna Townshend shares machine builder and system integrator news highlights from June 2024.By controldesign
…
continue reading
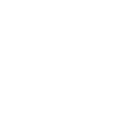
1
Alternatives to Administering and Running Apache Kafka
1:05:15
1:05:15
Play later
Play later
Lists
Like
Liked
1:05:15
View the show notes on the web: https://www.codingblocks.net/episode237 In the past couple of episodes, we'd gone over what Apache Kafka is and along the way we mentioned some of the pains of managing and running Kafka clusters on your own. In this episode, we discuss some of the ways you can offload those responsibilities and focus on writing stre…
…
continue reading
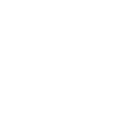
1
The changing role of PLCs in a connected plant
4:57
4:57
Play later
Play later
Lists
Like
Liked
4:57
In this episode of Control Intelligence, written by contributing editor Tobey Strauch, editor-in-chief Mike Bacidore discusses the future of PLCs in machinery used in a modular, connected factory or plant.By controldesign
…
continue reading
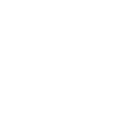
1
ANCA talks tech trends affecting machine builders
14:24
14:24
Play later
Play later
Lists
Like
Liked
14:24
In this episode of Control Intelligence, managing editor Anna Townshend discusses emerging technology and the introduction of autonomous manufacturing for CNC operation.By controldesign
…
continue reading
Topics, Partitions, and APIs oh my! This episode we're getting further into how Apache Kafka works and its use cases. Also, Allen is staying dry, Joe goes for broke, and Michael (eventually) gets on the right page. The full show notes are available on the website at https://www.codingblocks.net/episode236 News Thanks for the reviews! angingjellies …
…
continue reading
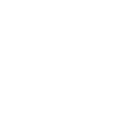
1
061 - OOUXing English Football with Will Poole
1:13:11
1:13:11
Play later
Play later
Lists
Like
Liked
1:13:11
Will Poole is Head of User Experience at Kyan, a digital product agency that specializes in strategy, design, and engineering, expertly crafting digital experiences to solve complex problems and drive innovation. In this episode of the podcast, Sophia and Will talk about Will's journey to finding OOUX, the challenges of bringing OOUX to Kyan, and u…
…
continue reading
In this episode of Control Intelligence, editor-in-chief Mike Bacidore explains how variable frequency drives provide efficiency, reliability and safety in manufacturing.By controldesign
…
continue reading
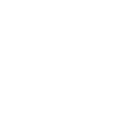
1
May 2024 industry news: expansions, partnerships and Automate
18:39
18:39
Play later
Play later
Lists
Like
Liked
18:39
In this episode of Control Intelligence, Anna Townshend shares machine builder and system integrator news highlights from the month of May.By controldesign
…
continue reading
We finally start talking about Apache Kafka! Also, Allen is getting acquainted with Aesop, Outlaw is killing clusters, and Joe is paying attention in drama class. The full show notes are available on the website at https://www.codingblocks.net/episode235 News Atlanta Dev Con is coming up, on September 7th, 2024 (www.atldevcon.com) Intro to Apache K…
…
continue reading
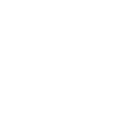
1
X-ray vision systems in the manufacturing industries
7:40
7:40
Play later
Play later
Lists
Like
Liked
7:40
In this episode of Control Intelligence, written by contributing editor Joey Stubbs, editor-in-chief Mike Bacidore discusses how x-ray vision systems have advanced defect detection.By controldesign
…
continue reading
Why can we not have slots in Livewire components?sBy Daniel Coulbourne & Caleb Porzio
…
continue reading