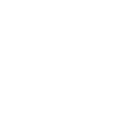
Go offline with the Player FM app!
S2E12: 'Building Powerful ML Models with Privacy & Ethics' with Katharine Jarmul (ThoughtWorks)
Manage episode 421035585 series 3407760
This week, I'm joined by Katharine Jarmul, Principal Data Scientist at Thoughtworks & author of the the forthcoming book, "Practical Data Privacy: Enhancing Privacy and Security in Data." Katharine began asking questions similar to those of today's ethical machine learning community as a university student working on her undergrad thesis during the war in Iraq. She focused that research on natural language processing and investigated the statistical differences between embedded & non-embedded reporters. In our conversation, we discuss ethical & secure machine learning approaches, threat modeling against adversarial attacks, the importance of distributed data setups, and what Katharine wants data scientists to know about privacy and ethical ML.
Katharine believes that we should never fall victim to a 'techno-solutionist' mindset where we believe that we can solve a deep societal problem simply with tech alone. However, by solving issues around privacy & consent with data collection, we can more easily address the challenges with ethical ML. In fact, ML research is finally beginning to broaden and include the intersections of law, privacy, and ethics. Katharine anticipates that data scientists will embrace PETs that facilitate data sharing in a privacy-preserving way; and, she evangelizes the un-normalization of sending ML data from one company to another.
Topics Covered:
- Katharine's motivation for writing a book on privacy for a data scientist audience and what she hopes readers will learn from it
- What areas must be addressed for ML to be considered ethical
- Overlapping AI/ML & Privacy goals
- Challenges with sharing data for analytics
- The need for data scientists to embrace PETs
- How PETs will likely mature across orgs over the next 2 years
- Katharine's & Debra's favorite PETs
- The importance of threat modeling ML models: discussing 'adversarial attacks' like 'model inversion' & 'membership inference' attacks
- Why companies that train LLMs must be accountable for the safety of their models
- New ethical approaches to data sharing
- Why scraping data off the Internet to train models is the harder, lazier, unethical way to train ML models
Resources Mentioned:
- Pre-order the forthcoming book: "Practical Data Privacy"
- Subscribe to Katharine’s newsletter: Probably Private
Guest Info:
Privado.ai
Privacy assurance at the speed of product development. Get instant visibility w/ privacy code scans.
Shifting Privacy Left Media
Where privacy engineers gather, share, & learn
Buzzsprout - Launch your podcast
Disclaimer: This post contains affiliate links. If you make a purchase, I may receive a commission at no extra cost to you.
Copyright © 2022 - 2024 Principled LLC. All rights reserved.
Chapters
1. S2E12: 'Building Powerful ML Models with Privacy & Ethics' with Katharine Jarmul (ThoughtWorks) (00:00:00)
2. Introducing Katharine Jarmul, Principal Data Scientist at ThoughtWorks, where she shares her data science & privacy origin story (00:00:27)
3. Katharine describes why she was inspired to write the book, Practical Data Privacy (00:04:13)
4. Katharine explains why she wrote a book on privacy for a data scientist audience and what she hopes readers will learn from it (00:10:42)
5. Katharine & Debra discuss what areas must be addressed for machine learning to be considered ethical (00:13:56)
6. Katherine & Debra discuss the overlapping AI/ML & Privacy goals of: fairness, accountability, & transparency as well as overlapping research (00:18:38)
7. Derbra & Katharine discuss challenges with sharing data for analytics; the need for data scientists to embrace PETs; and why we should stop normalizing the idea of sending data to another company...ever (00:21:31)
8. Debra shares her belief that there soon will be an 'explosion of PET' uses and cites a new report from The United Nations (00:24:46)
9. Katharine shares her thoughts on how PETs are going to mature across orgs over the next 2 years (00:25:58)
10. Debra & Katharine share each of their favorite PETs - Debra discusses self-sovereign identity and Katharine talks up Secure Multi-Party Computation (00:28:14)
11. Debra discusses her renewed sense of optimism about privacy and technology due the research, implementation, & standardization of PETs (00:32:08)
12. Katharine discusses the need to threat model ML models, discussing 'adversarial attacks,' including: 'model inversion' attacks & 'membership inference' attacks (00:34:35)
13. Debra & Katharine discuss the issue of lack of consent when it comes to training LLMs (00:42:52)
14. Katharine explains why companies that train LLMs must be accountable for the safety of their models, never putting that onus on users (00:47:57)
15. Katharine explains why scraping data off the Internet to train models is actually the harder, lazier way to train ML models (00:49:39)
16. Katharine plugs her ethical data science newsletter, 'Probably Private' (00:52:12)
62 episodes
Manage episode 421035585 series 3407760
This week, I'm joined by Katharine Jarmul, Principal Data Scientist at Thoughtworks & author of the the forthcoming book, "Practical Data Privacy: Enhancing Privacy and Security in Data." Katharine began asking questions similar to those of today's ethical machine learning community as a university student working on her undergrad thesis during the war in Iraq. She focused that research on natural language processing and investigated the statistical differences between embedded & non-embedded reporters. In our conversation, we discuss ethical & secure machine learning approaches, threat modeling against adversarial attacks, the importance of distributed data setups, and what Katharine wants data scientists to know about privacy and ethical ML.
Katharine believes that we should never fall victim to a 'techno-solutionist' mindset where we believe that we can solve a deep societal problem simply with tech alone. However, by solving issues around privacy & consent with data collection, we can more easily address the challenges with ethical ML. In fact, ML research is finally beginning to broaden and include the intersections of law, privacy, and ethics. Katharine anticipates that data scientists will embrace PETs that facilitate data sharing in a privacy-preserving way; and, she evangelizes the un-normalization of sending ML data from one company to another.
Topics Covered:
- Katharine's motivation for writing a book on privacy for a data scientist audience and what she hopes readers will learn from it
- What areas must be addressed for ML to be considered ethical
- Overlapping AI/ML & Privacy goals
- Challenges with sharing data for analytics
- The need for data scientists to embrace PETs
- How PETs will likely mature across orgs over the next 2 years
- Katharine's & Debra's favorite PETs
- The importance of threat modeling ML models: discussing 'adversarial attacks' like 'model inversion' & 'membership inference' attacks
- Why companies that train LLMs must be accountable for the safety of their models
- New ethical approaches to data sharing
- Why scraping data off the Internet to train models is the harder, lazier, unethical way to train ML models
Resources Mentioned:
- Pre-order the forthcoming book: "Practical Data Privacy"
- Subscribe to Katharine’s newsletter: Probably Private
Guest Info:
Privado.ai
Privacy assurance at the speed of product development. Get instant visibility w/ privacy code scans.
Shifting Privacy Left Media
Where privacy engineers gather, share, & learn
Buzzsprout - Launch your podcast
Disclaimer: This post contains affiliate links. If you make a purchase, I may receive a commission at no extra cost to you.
Copyright © 2022 - 2024 Principled LLC. All rights reserved.
Chapters
1. S2E12: 'Building Powerful ML Models with Privacy & Ethics' with Katharine Jarmul (ThoughtWorks) (00:00:00)
2. Introducing Katharine Jarmul, Principal Data Scientist at ThoughtWorks, where she shares her data science & privacy origin story (00:00:27)
3. Katharine describes why she was inspired to write the book, Practical Data Privacy (00:04:13)
4. Katharine explains why she wrote a book on privacy for a data scientist audience and what she hopes readers will learn from it (00:10:42)
5. Katharine & Debra discuss what areas must be addressed for machine learning to be considered ethical (00:13:56)
6. Katherine & Debra discuss the overlapping AI/ML & Privacy goals of: fairness, accountability, & transparency as well as overlapping research (00:18:38)
7. Derbra & Katharine discuss challenges with sharing data for analytics; the need for data scientists to embrace PETs; and why we should stop normalizing the idea of sending data to another company...ever (00:21:31)
8. Debra shares her belief that there soon will be an 'explosion of PET' uses and cites a new report from The United Nations (00:24:46)
9. Katharine shares her thoughts on how PETs are going to mature across orgs over the next 2 years (00:25:58)
10. Debra & Katharine share each of their favorite PETs - Debra discusses self-sovereign identity and Katharine talks up Secure Multi-Party Computation (00:28:14)
11. Debra discusses her renewed sense of optimism about privacy and technology due the research, implementation, & standardization of PETs (00:32:08)
12. Katharine discusses the need to threat model ML models, discussing 'adversarial attacks,' including: 'model inversion' attacks & 'membership inference' attacks (00:34:35)
13. Debra & Katharine discuss the issue of lack of consent when it comes to training LLMs (00:42:52)
14. Katharine explains why companies that train LLMs must be accountable for the safety of their models, never putting that onus on users (00:47:57)
15. Katharine explains why scraping data off the Internet to train models is actually the harder, lazier way to train ML models (00:49:39)
16. Katharine plugs her ethical data science newsletter, 'Probably Private' (00:52:12)
62 episodes
All episodes
×Welcome to Player FM!
Player FM is scanning the web for high-quality podcasts for you to enjoy right now. It's the best podcast app and works on Android, iPhone, and the web. Signup to sync subscriptions across devices.