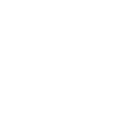
Go offline with the Player FM app!
S2E8: Leveraging Federated Learning for Input Privacy with Victor Platt
Manage episode 421035588 series 3407760
Victor Platt is a Senior AI Security and Privacy Strategist who previously served as Head of Security and Privacy for privacy tech company, Integrate.ai. Victor was formerly a founding member of the Risk AI Team with Omnia AI, Deloitt’s artificial intelligence practice in Canada. He joins today to discuss privacy enhancing technologies (PETs) that are shaping industries around the world, with a focus on federated learning.
---------
Thank you to our sponsor, Privado, the developer-friendly privacy platform
---------
Victor views PETs as functional requirements and says they shouldn’t be buried in your design document as nonfunctional obligations. In his work, he has found key gaps where organizations were only doing “security for security’s sake.” Rather, he believes organizations should be thinking about it at the forefront. Not only that, we should all be getting excited about it because we all have a stake in privacy.
With federated learning, you have the tools available to train ML models on large data sets with precision at scale without risking user privacy. In this conversation, Victor demystifies what federated learning is, describes the 2 different types: at the edge and across data silos, and explains how it works and how it compares to traditional machine learning.We deep dive into how an organization knows when to use federated learning, with specific advice for developers and data scientists as they implement it into their organizations.
Topics Covered:
- What 'federated learning' is and how it compares to traditional machine learning
- When an organization should use vertical federated learning vs horizontal federated learning, or instead a hybrid version
- A key challenge in 'transfer learning': knowing whether two data sets are related to each other and techniques to overcome this, like 'private set intersection'
- How the future of technology will be underpinned by a 'constellation of PETs'
- The distinction between 'input privacy' vs. 'output privacy'
- Different kinds of federated learning with use case examples
- Where the responsibility for adding PETs lies within an organization
- The key barriers to adopting federated learning and other PETs within different industries and use cases
- How to move the needle on data privacy when it comes to legislation and regulation
Resources Mentioned:
- Take this outstanding, free class from OpenMined: Our Privacy Opportunity
Guest Info:
- Follow Victor on LinkedIn
Follow the SPL Show:
Privado.ai
Privacy assurance at the speed of product development. Get instant visibility w/ privacy code scans.
Shifting Privacy Left Media
Where privacy engineers gather, share, & learn
Buzzsprout - Launch your podcast
Disclaimer: This post contains affiliate links. If you make a purchase, I may receive a commission at no extra cost to you.
Copyright © 2022 - 2024 Principled LLC. All rights reserved.
Chapters
1. S2E8: Leveraging Federated Learning for Input Privacy with Victor Platt (00:00:00)
2. Introducing Victor Platt (00:00:27)
3. Victor's 'origin story' and how he got interested in PETs like 'federated learning' (00:01:32)
4. Victor describes what 'federated learning' is and how it compares to traditional machine learning (00:04:49)
5. Victor explains the use of federated learning 'at the edge' & example use cases (00:06:04)
6. Victor explains federated learning 'across silos' & example use cases (00:07:57)
7. Victor explains when an org would consider using vertical vs. horizontal federated learning or a hybrid of both (00:10:48)
8. Victor outlines a key challenge in 'transfer learning': knowing whether two data sets are related to each other. He also explains how 'private set intersection' techniques can help blindly identify common rows between data sets (00:12:36)
9. Victor describes the two paths to when you should consider federated learning for your use case (00:15:09)
10. Victor shares his excitement for the future of technology, which he believes will be underpinned by a 'constellation of PETs'. He also distinguishes between PETs that assist with 'input privacy' vs. 'output privacy' (00:18:04)
11. Victor shares his view on where the responsibility for leveraging PETs lies within an org (00:20:58)
12. Victor describes key barriers to adoption of federated learning & other PETs within different industries (00:24:02)
13. Victor shares how we can move the needle on updating laws that have antiquated approaches to anonymity / 'de-identification' (e.g. HIPAA) and to include the use of new PETs (00:27:41)
14. Debra & Victor discuss standards in development related to PETs like federated learning, differential privacy & homomorphic encryption (00:34:14)
15. Victor shares his advice for developers and data scientists as they implement privacy by design into their organizations. He recommends taking OpenMined's (free) course: 'Our Privacy Opportunity' (00:36:06)
62 episodes
Manage episode 421035588 series 3407760
Victor Platt is a Senior AI Security and Privacy Strategist who previously served as Head of Security and Privacy for privacy tech company, Integrate.ai. Victor was formerly a founding member of the Risk AI Team with Omnia AI, Deloitt’s artificial intelligence practice in Canada. He joins today to discuss privacy enhancing technologies (PETs) that are shaping industries around the world, with a focus on federated learning.
---------
Thank you to our sponsor, Privado, the developer-friendly privacy platform
---------
Victor views PETs as functional requirements and says they shouldn’t be buried in your design document as nonfunctional obligations. In his work, he has found key gaps where organizations were only doing “security for security’s sake.” Rather, he believes organizations should be thinking about it at the forefront. Not only that, we should all be getting excited about it because we all have a stake in privacy.
With federated learning, you have the tools available to train ML models on large data sets with precision at scale without risking user privacy. In this conversation, Victor demystifies what federated learning is, describes the 2 different types: at the edge and across data silos, and explains how it works and how it compares to traditional machine learning.We deep dive into how an organization knows when to use federated learning, with specific advice for developers and data scientists as they implement it into their organizations.
Topics Covered:
- What 'federated learning' is and how it compares to traditional machine learning
- When an organization should use vertical federated learning vs horizontal federated learning, or instead a hybrid version
- A key challenge in 'transfer learning': knowing whether two data sets are related to each other and techniques to overcome this, like 'private set intersection'
- How the future of technology will be underpinned by a 'constellation of PETs'
- The distinction between 'input privacy' vs. 'output privacy'
- Different kinds of federated learning with use case examples
- Where the responsibility for adding PETs lies within an organization
- The key barriers to adopting federated learning and other PETs within different industries and use cases
- How to move the needle on data privacy when it comes to legislation and regulation
Resources Mentioned:
- Take this outstanding, free class from OpenMined: Our Privacy Opportunity
Guest Info:
- Follow Victor on LinkedIn
Follow the SPL Show:
Privado.ai
Privacy assurance at the speed of product development. Get instant visibility w/ privacy code scans.
Shifting Privacy Left Media
Where privacy engineers gather, share, & learn
Buzzsprout - Launch your podcast
Disclaimer: This post contains affiliate links. If you make a purchase, I may receive a commission at no extra cost to you.
Copyright © 2022 - 2024 Principled LLC. All rights reserved.
Chapters
1. S2E8: Leveraging Federated Learning for Input Privacy with Victor Platt (00:00:00)
2. Introducing Victor Platt (00:00:27)
3. Victor's 'origin story' and how he got interested in PETs like 'federated learning' (00:01:32)
4. Victor describes what 'federated learning' is and how it compares to traditional machine learning (00:04:49)
5. Victor explains the use of federated learning 'at the edge' & example use cases (00:06:04)
6. Victor explains federated learning 'across silos' & example use cases (00:07:57)
7. Victor explains when an org would consider using vertical vs. horizontal federated learning or a hybrid of both (00:10:48)
8. Victor outlines a key challenge in 'transfer learning': knowing whether two data sets are related to each other. He also explains how 'private set intersection' techniques can help blindly identify common rows between data sets (00:12:36)
9. Victor describes the two paths to when you should consider federated learning for your use case (00:15:09)
10. Victor shares his excitement for the future of technology, which he believes will be underpinned by a 'constellation of PETs'. He also distinguishes between PETs that assist with 'input privacy' vs. 'output privacy' (00:18:04)
11. Victor shares his view on where the responsibility for leveraging PETs lies within an org (00:20:58)
12. Victor describes key barriers to adoption of federated learning & other PETs within different industries (00:24:02)
13. Victor shares how we can move the needle on updating laws that have antiquated approaches to anonymity / 'de-identification' (e.g. HIPAA) and to include the use of new PETs (00:27:41)
14. Debra & Victor discuss standards in development related to PETs like federated learning, differential privacy & homomorphic encryption (00:34:14)
15. Victor shares his advice for developers and data scientists as they implement privacy by design into their organizations. He recommends taking OpenMined's (free) course: 'Our Privacy Opportunity' (00:36:06)
62 episodes
All episodes
×Welcome to Player FM!
Player FM is scanning the web for high-quality podcasts for you to enjoy right now. It's the best podcast app and works on Android, iPhone, and the web. Signup to sync subscriptions across devices.