Go offline with the Player FM app!
Being Data Driven At Stripe With Trino And Iceberg
Manage episode 423933376 series 3449056
Summary
Stripe is a company that relies on data to power their products and business. To support that functionality they have invested in Trino and Iceberg for their analytical workloads. In this episode Kevin Liu shares some of the interesting features that they have built by combining those technologies, as well as the challenges that they face in supporting the myriad workloads that are thrown at this layer of their data platform.
Announcements
- Hello and welcome to the Data Engineering Podcast, the show about modern data management
- Data lakes are notoriously complex. For data engineers who battle to build and scale high quality data workflows on the data lake, Starburst is an end-to-end data lakehouse platform built on Trino, the query engine Apache Iceberg was designed for, with complete support for all table formats including Apache Iceberg, Hive, and Delta Lake. Trusted by teams of all sizes, including Comcast and Doordash. Want to see Starburst in action? Go to dataengineeringpodcast.com/starburst and get $500 in credits to try Starburst Galaxy today, the easiest and fastest way to get started using Trino.
- Your host is Tobias Macey and today I'm interviewing Kevin Liu about his use of Trino and Iceberg for Stripe's data lakehouse
Interview
- Introduction
- How did you get involved in the area of data management?
- Can you describe what role Trino and Iceberg play in Stripe's data architecture?
- What are the ways in which your job responsibilities intersect with Stripe's lakehouse infrastructure?
- What were the requirements and selection criteria that led to the selection of that combination of technologies?
- What are the other systems that feed into and rely on the Trino/Iceberg service?
- what kinds of questions are you answering with table metadata
- what use case/team does that support
- comparative utility of iceberg REST catalog
- What are the shortcomings of Trino and Iceberg?
- What are the most interesting, innovative, or unexpected ways that you have seen Iceberg/Trino used?
- What are the most interesting, unexpected, or challenging lessons that you have learned while working on Stripe's data infrastructure?
- When is a lakehouse on Trino/Iceberg the wrong choice?
- What do you have planned for the future of Trino and Iceberg at Stripe?
Contact Info
Parting Question
- From your perspective, what is the biggest gap in the tooling or technology for data management today?
Closing Announcements
- Thank you for listening! Don't forget to check out our other shows. Podcast.__init__ covers the Python language, its community, and the innovative ways it is being used. The Machine Learning Podcast helps you go from idea to production with machine learning.
- Visit the site to subscribe to the show, sign up for the mailing list, and read the show notes.
- If you've learned something or tried out a project from the show then tell us about it! Email hosts@dataengineeringpodcast.com with your story.
Links
- Trino
- Iceberg
- Stripe
- Spark
- Redshift
- Hive Metastore
- Python Iceberg
- Python Iceberg REST Catalog
- Trino Metadata Table
- Flink
- Tabular
- Delta Table
- Databricks Unity Catalog
- Starburst
- AWS Athena
- Kevin Trinofest Presentation
- Alluxio
- Parquet
- Hudi
- Trino Project Tardigrade
- Trino On Ice
The intro and outro music is from The Hug by The Freak Fandango Orchestra / CC BY-SA
Sponsored By:
- Starburst: 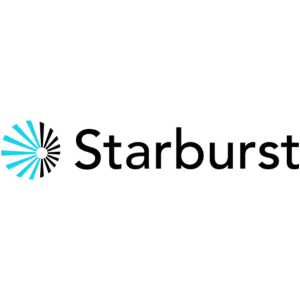 This episode is brought to you by Starburst - an end-to-end data lakehouse platform for data engineers who are battling to build and scale high quality data pipelines on the data lake. Powered by Trino, the query engine Apache Iceberg was designed for, Starburst is an open platform with support for all table formats including Apache Iceberg, Hive, and Delta Lake. Trusted by the teams at Comcast and Doordash, Starburst delivers the adaptability and flexibility a lakehouse ecosystem promises, while providing a single point of access for your data and all your data governance allowing you to discover, transform, govern, and secure all in one place. Want to see Starburst in action? Try Starburst Galaxy today, the easiest and fastest way to get started using Trino, and get $500 of credits free. Go to [dataengineeringpodcast.com/starburst](https://www.dataengineeringpodcast.com/starburst)
438 episodes
Manage episode 423933376 series 3449056
Summary
Stripe is a company that relies on data to power their products and business. To support that functionality they have invested in Trino and Iceberg for their analytical workloads. In this episode Kevin Liu shares some of the interesting features that they have built by combining those technologies, as well as the challenges that they face in supporting the myriad workloads that are thrown at this layer of their data platform.
Announcements
- Hello and welcome to the Data Engineering Podcast, the show about modern data management
- Data lakes are notoriously complex. For data engineers who battle to build and scale high quality data workflows on the data lake, Starburst is an end-to-end data lakehouse platform built on Trino, the query engine Apache Iceberg was designed for, with complete support for all table formats including Apache Iceberg, Hive, and Delta Lake. Trusted by teams of all sizes, including Comcast and Doordash. Want to see Starburst in action? Go to dataengineeringpodcast.com/starburst and get $500 in credits to try Starburst Galaxy today, the easiest and fastest way to get started using Trino.
- Your host is Tobias Macey and today I'm interviewing Kevin Liu about his use of Trino and Iceberg for Stripe's data lakehouse
Interview
- Introduction
- How did you get involved in the area of data management?
- Can you describe what role Trino and Iceberg play in Stripe's data architecture?
- What are the ways in which your job responsibilities intersect with Stripe's lakehouse infrastructure?
- What were the requirements and selection criteria that led to the selection of that combination of technologies?
- What are the other systems that feed into and rely on the Trino/Iceberg service?
- what kinds of questions are you answering with table metadata
- what use case/team does that support
- comparative utility of iceberg REST catalog
- What are the shortcomings of Trino and Iceberg?
- What are the most interesting, innovative, or unexpected ways that you have seen Iceberg/Trino used?
- What are the most interesting, unexpected, or challenging lessons that you have learned while working on Stripe's data infrastructure?
- When is a lakehouse on Trino/Iceberg the wrong choice?
- What do you have planned for the future of Trino and Iceberg at Stripe?
Contact Info
Parting Question
- From your perspective, what is the biggest gap in the tooling or technology for data management today?
Closing Announcements
- Thank you for listening! Don't forget to check out our other shows. Podcast.__init__ covers the Python language, its community, and the innovative ways it is being used. The Machine Learning Podcast helps you go from idea to production with machine learning.
- Visit the site to subscribe to the show, sign up for the mailing list, and read the show notes.
- If you've learned something or tried out a project from the show then tell us about it! Email hosts@dataengineeringpodcast.com with your story.
Links
- Trino
- Iceberg
- Stripe
- Spark
- Redshift
- Hive Metastore
- Python Iceberg
- Python Iceberg REST Catalog
- Trino Metadata Table
- Flink
- Tabular
- Delta Table
- Databricks Unity Catalog
- Starburst
- AWS Athena
- Kevin Trinofest Presentation
- Alluxio
- Parquet
- Hudi
- Trino Project Tardigrade
- Trino On Ice
The intro and outro music is from The Hug by The Freak Fandango Orchestra / CC BY-SA
Sponsored By:
- Starburst: 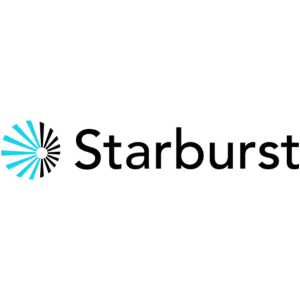 This episode is brought to you by Starburst - an end-to-end data lakehouse platform for data engineers who are battling to build and scale high quality data pipelines on the data lake. Powered by Trino, the query engine Apache Iceberg was designed for, Starburst is an open platform with support for all table formats including Apache Iceberg, Hive, and Delta Lake. Trusted by the teams at Comcast and Doordash, Starburst delivers the adaptability and flexibility a lakehouse ecosystem promises, while providing a single point of access for your data and all your data governance allowing you to discover, transform, govern, and secure all in one place. Want to see Starburst in action? Try Starburst Galaxy today, the easiest and fastest way to get started using Trino, and get $500 of credits free. Go to [dataengineeringpodcast.com/starburst](https://www.dataengineeringpodcast.com/starburst)
438 episodes
All episodes
×Welcome to Player FM!
Player FM is scanning the web for high-quality podcasts for you to enjoy right now. It's the best podcast app and works on Android, iPhone, and the web. Signup to sync subscriptions across devices.